What is AI bias?
Artificial intelligence bias refers to situations when AI produces biased, incorrect, or incomplete conclusions due to gaps in training and data availability. Because it is created based on machine learning about data, it requires diversified training and data to produce unbiased results.
One example is generative AI, which requires large language models (LLMs) and more data than any one institution can provide. To work, it needs enough data to allow AI to learn to create content as a human would, whether that be written words in natural language, an image, or software. But, there are often significant holes in the data that not only limit the functionality but that also contribute to bias.
How does bias affect AI?
To understand AI bias, we first need to understand how generative AI deals with incomplete information sets. AI works on probabilities of what it knows. When there are gaps in generative AI’s knowledge, it may fill the logical gaps, not unlike how humans do; in short, generative AI can “hallucinate” or invent things by using information with much lower probabilities of being correct. Equally as concerning, AI can sometimes completely ignore the areas where it doesn’t have enough data. This often leads to what we would call AI bias. The AI wasn’t trained on enough of a certain type of data, so it ignored a population.
An example of this would be if AI created images with people with lighter skin but not with darker skin – because it wasn’t trained on enough people images with darker skin.
Example: I prompted Starry AI with “4-6 people digging looking for treasure.” All of the images, like the attached, were of lighter-skinned people.
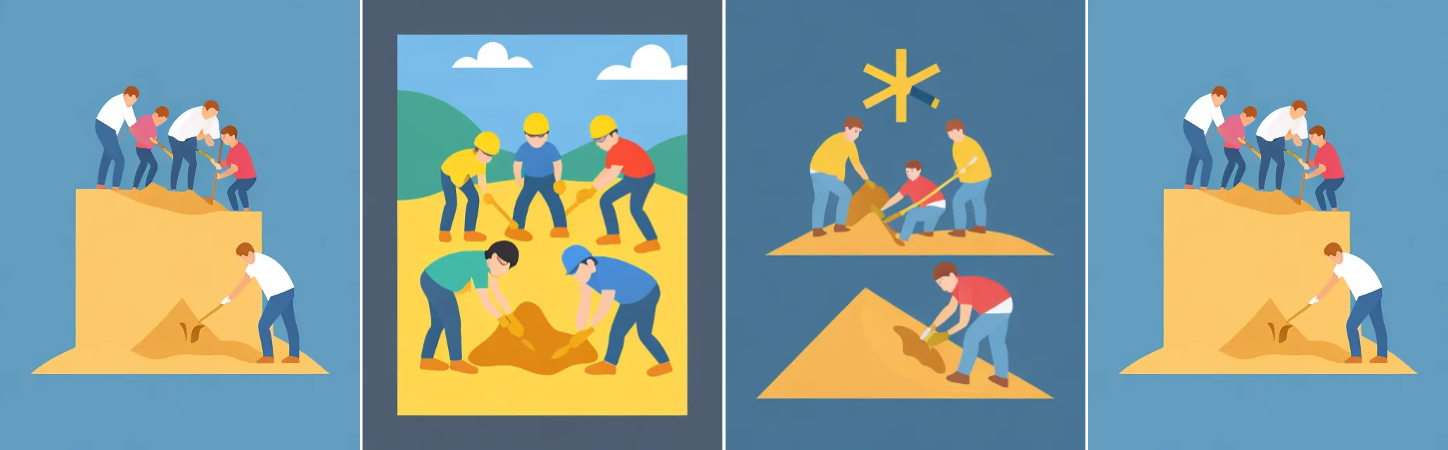
Bias can easily ignore a population – even unintentionally. The machine was not trained to recognize that populations should be diverse, so it doesn’t automatically represent diverse populations.
How big of a concern is AI bias?
AI bias has incredible implications – some of which can and will be long-term if not circumvented. For example, what if we are using AI to help us determine housing values for mortgage appraisals, and it creates an accidental redlining scenario because it wasn’t trained properly with diverse data? These problems could affect people for generations, just as redlining policies in the FHA from the 1930s still affect neighborhoods and the wealth of certain populations today.
How can AI bias be mitigated?
Mitigating AI bias is primarily done through testing and training with diverse data and building systems with a diverse set of people. First, the data we use needs to be intentionally diverse. We have to try things that are outside of our own normative cultural behaviors and train systems to understand that.
When I worked at IBM in the early 2000s, IBM researchers would give free lunches to as many of the 100,000+ U.S. employees as they could, with the goal of helping their speech recognition training. While 100,000+ people might sound like a great data set, the research team knew they had to get people from different socio-economic classes and from different regions where IBM did not have employees. It wasn’t just about the numbers, but the diversity of the data set –and enough diversity to properly train it.
Diverse teams work through diversity problems best. Several years ago, when BUILT created a kiosk system that included facial recognition, it was because we had a diverse team creating and testing the product that we realized the facial recognition service we were using struggled with darker skin if we didn’t illuminate the person’s face better. If we had not had a diverse team, we would have never known.
AI bias is much the same. It reflects human bias, even unconsciously, which is why utilizing diverse teams to test and train systems through diverse data sets is imperative.
Will there ever be a day when there is no AI bias?
There will never be a day we do not have to worry about AI bias. Just as people using machine learning to do speech recognition 20 years ago had to worry about it, current and future generations will need to actively address bias within AI as well. Without careful attention, we can easily use data and training that support our implicit biases, which is why taking proactive steps to mitigate and prevent AI bias will always be essential.
Interested in learning more about how BUILT’s digital transformation experts can take your business to the next level? Connect today: https://www.builtglobal.com/contact